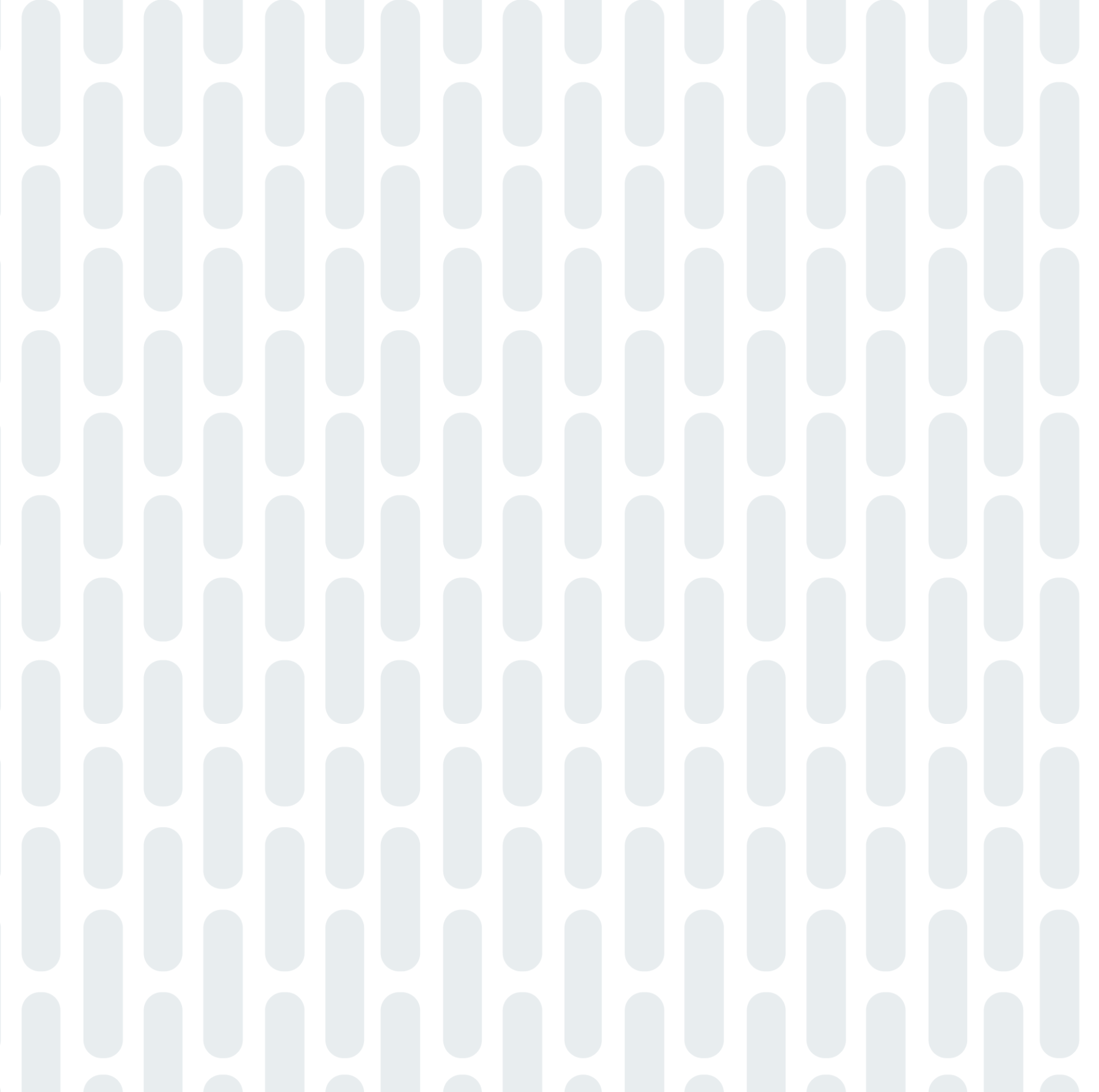
Reprints
Utilizing the Microbiota and Machine Learning Algorithms To Assess Risk of Salmonella Contamination in Poultry Rinsate
Journal of Food Protection
Bolinger H., Tran D., Harary K., Paoli G.C., Guron G.K.P., Namazi H. & Khaksar R.
Abstract
Traditional microbiological testing methods are slow, and many molecular-based techniques rely on culture-based enrichment to overcome low limits of detection. Recent advancements in sequencing technologies may make it possible to utilize machine learning to identify patterns in microbiome data to potentially predict the presence or absence of pathogens. In this study, 299 poultry rinsate samples from various points in the processing chain were analyzed to determine if microbiota could inform about a sample’s risk for containing Salmonella. Samples were culture confirmed as Salmonella positive or negative following modified U.S. Department of Agriculture Microbiological Laboratory Guidebook protocols. The culture confirmation result was used as a reference to compare with 16S sequencing data. Prechill samples tested positive (71 of 82) at a higher frequency than postchill samples (30 of 217) and contained greater microbial diversity. Due to the larger sample size, postchill samples were analyzed more thoroughly. Analysis of variance identified a significant effect of chilling on the number of genera (P < 0.001), but analysis of similarities failed to provide evidence for microbial dissimilarity between pre- and postchill samples (P = 0.001, R = 0.443). Various machine learning models were trained by using postchill samples to predict if a sample contained Salmonella on the basis of the samples’ microbiota preenrichment. The optimal model was a random forest–based model with a performance as follows: accuracy (88%), sensitivity (85%), and specificity (90%). Although the algorithms described in this article are prototypes, these risk-based algorithms demonstrate the potential and need for further studies to provide insight alongside diagnostic tests. Combining risk-based information with diagnostic tools can help poultry processors make informed decisions to help identify and prevent the spread of Salmonella. These data add to the growing body of literature exploring novel ways to utilize microbiome data for predictive food safety.
To view the full article, please click here.